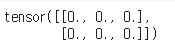
2행 3열 0으로 초기화된 Tensor만들기 ( 배열만들기 )
torch import 해야됨
# torch.zeros(2,3) ---> [[0,0,0], ..... [0,0,0]]
x = torch.zeros(2,3)
x
2행 3열 1로 초기화된 Tensor만들기
# torch.ones(2,3) --> [[1,1,1], .....[1,1,1]]
x = torch.ones(2,3)
x
1차원 배열 0~3까지 0.5씩 증가하는 Tensor만들기
1차원 배열도 Tensor임
# torch.arange(start, end, step=1)
x = torch.arange(0, 3, step=0.5)
x
- Tensor Data Type
2행 3열 float 자료형 tensor 만들기
# torch.FloatTensor(size or list)
x = torch.FloatTensor(2, 3)
x
list를 사용해서 float 자료형 tensor 만들기
x = torch.FloatTensor([12,35])
x
위에서 쓴 Float Tensor x를 IntTensor로 바꿈
# tensor.type_as(tensor_type)
# 기존 텐서의 값의 자료형을 바꿀 때 사용
x = x.type_as(torch.IntTensor())
x
- Numpy to Tensor, Tensor to Numpy
buffer 변수에 값을 리스트로 넣으면 numpy가 이 값들로 배열을 만들어줌
import numpy as np
# torch.from_numpy(ndarray) --> Tensor
x1 = np.ndarray(shape=(2,3), dtype=int, buffer=np.array([1,2,3,4,5,6]))
x2 = torch.from_numpy(x1)
x1,x2
자료형이 바뀐거 확인
다시 Tensor를 np.array로
# torch.numpy() --> ndarray
x3 = x2.numpy()
x3
- Tensor on CPU & GPU
2행 3열 Tensor 만들기
x = torch.FloatTensor([[1,2,3],[4,5,6]])
x
cuda를 쓰면 gpu를 씀
x_gpu = x.cuda()
x_gpu
- Tensor Size
# tensor.size() --> 인덱스값이
x = torch.FloatTensor(10, 12, 3, 3)
x
4차원 Tensor 생성
x.size()
사이즈 확인
- Indexing, Slicing, Joining
- Indexing
# torch.index_select(input, dim, index)
x = torch.rand(4,3)
out = torch.index_select(x, 0, torch.LongTensor([0,3]))
x, out
# python indexing 사용 가능
x[:, 0], x[0, :], x[0:2, 0:2]
x[:, 0] 0열 전부 select
x[ 0, : ] 0행 select
x[0:2, 0:2] 0~2행 , 0~2열 select
# torch.masked_select(input, mask)
x = torch.randn(2,3)
mask = torch.BoolTensor([[0,0,1],[0, 1, 0]])
out = torch.masked_select(x,mask)
out
마스크 씌워서 원하는곳 값만 추출
- Joining
# torch.cat(seq, dim=0)
x = torch.FloatTensor([[1,2,3], [4,5,6]])
y = torch.FloatTensor([[-1,-2,-3], [-4,-5,-6]])
z1 = torch.cat([x, y], dim=0) # dim=0 (행), 아래에 합쳐짐
x,y,z1
dim이 0이라 아래에 합침.
dim이 1이면
z2 = torch.cat([x, y], dim=1) # dim=1 (열), 옆에 합쳐짐
2행 3열 4개를 합쳐서 4면 2행 3열로 만들기
# torch.stack(sequence, dim=0) --> 새로운 차원으로 추가
x = torch.FloatTensor([[1,2,3], [4,5,6]]) # (2,3) shape 2행 3열 2차원
x_stack = torch.stack([x, x, x, x], dim=0) # (4, 2, 3) 4면 2행 3열 3차원
x, x_stack
x
x_stack
- Slicing
위에서 쓴 z1을 x_1, x_2 두개로 분할
# torch.chunk(tensor, chunks, dim=0)
x_1, x_2 = torch.chunk(z1, 2, dim =0) # dim=0이면 행을 2개로 분할 (여기서 2 는 chuck갯수)
x_1, x_2
z1을 y_1, y_2, y_3 세개로 열로 분할
y_1, y_2, y_3 = torch.chunk(z1, 3, dim =1) # dim =1 이면 열을 3개로 분할
y_1, y_2, y_3
split을 사용한 slicing
# torch.split(tensor, split_size, dim=0)
x1, x2 = torch.split(z1, 2, dim=0)
y1 = torch.split(z1, 2, dim=1) # 3개 열을 2개로 분리한 경우
z1, x1, x2, y1
원본 z1
x1, x2
y1 => tuple형태로 묶어서 분리됨
squeezing
x1 = torch.FloatTensor(10, 1, 3, 14)
# 차원(dimension) 의 개념
# 10 : batch size (데이터 한 묶음당 들어있는 데이터(x,y)쌍의 갯수)
# 1 : channel size
# 3 : with image size
# 14 : height image size
x2 = torch.squeeze(x1)
x1.size(), x2.size()
squeeze를 하면 차원이 하나 줄음
반대개념인 unsqueeze도 있음. 숫자가 1인 차원을 하나 추가
# torch.unsqueeze(input, dim=None) ---> 숫자 1을 추가
x1 = torch.FloatTensor(10, 3, 4)
x2 = torch.unsqueeze(x1, dim=0) # dim=0 이면 첫번째 위치에 추가
x1.size(), x2.size()
첫번째 위치에 차원이 하나 늘어난거 확인
- Tensor 초기화
x1 = init.uniform(torch.FloatTensor(3,4), a=0, b=9) # a에서 b사이의 값으로 초기화
x2 = init.normal(torch.FloatTensor(3,4), std=0.2) # 표준편차가 0.2 적용 초기화
x3 = init.constant(torch.FloatTensor(3,4), 3.145) # 지정된 상수값으로 초기화
x1, x2, x3
- Tensor 연산
- Arithmetic operations (산술연산)
# torch.add()
x1 = torch.FloatTensor([[1,2,3],[4,5,6]])
x2 = torch.FloatTensor([[1,2,3],[4,5,6]])
add = torch.add(x1, x2)
x1, x2, add, x1 + x2, x1-x2
-벡터화연산함수 지원
+로 한거와 add함수를 쓴것 결과가 같음
x1 = torch.FloatTensor([[1,2,3],[4,5,6]])
x2 = torch.add(x1,10)
x1, x2, x1 + 10
-곱하기
# torch.mul() 곱하기
x1 = torch.FloatTensor([[1,2,3],[4,5,6]])
x2 = torch.FloatTensor([[1,2,3],[4,5,6]])
x3 = torch.mul(x1,x2)
x3, x1 * x2
-나누기
# torch.div() 나누기
x1 = torch.FloatTensor([[1,2,3],[4,5,6]])
x2 = torch.div(x1, 5)
x1, x2, x1 /5
-제곱
# torch.pow(input, exponent)
x1 = torch.FloatTensor([[1,2,3],[4,5,6]])
x1, torch.pow(x1, 2), x1**2
모두 함수를쓰나 기호를쓰나 결과는 같다.
-지수함수
# torch.exp(tensor, out=None)
x1 = torch.FloatTensor([[1,2,3],[4,5,6]])
out = torch.exp(x1)
x1,out
-로그함수
# torch.log(input, out=None) 자연로그 계산
x1 = torch.FloatTensor([[1,2,3],[4,5,6]])
out = torch.log(x1)
x1,out
- Matrix operations (행렬연산)
-대학교때 죽어라 하던 행렬곱 mm
# torch.mm(mat1, mat2) --> matrix multiplication (행렬곱)
x1 = torch.FloatTensor(3,4)
x2 = torch.FloatTensor(4,5)
out = torch.mm(x1,x2)
x1, x2, out
3행 4열 * 4행 5열이니 결과는 3행 5열이다
-bmm 행렬곱
# torch.bmm(batch1, batch2) --> batch 행렬곱 연산
# 맨 앞의 batch 는 차원을 유지하면서, 뒤 요소들의 행렬곱 연산함
x1 = torch.FloatTensor(10, 3, 4)
x2 = torch.FloatTensor(10, 4, 5)
out = torch.bmm(x1, x2)
out.size()
앞의 차원은 두고 뒤의 차원이 바뀜
-내적구하기
# torch.dot(tensor1, tensor2) : 벡터의 내적을 구함
x1 = torch.FloatTensor([1,2,3,4])
x2 = torch.FloatTensor([2,3,4,5])
torch.dot(x1, x2)
곱해서 다 더하는거 인듯
-전치연산
# torch.t(matrix) : transposed matrix (텐서의 전치 연산)
# 행과 열을 바꿈
x1 = torch.FloatTensor(3,4)
x1, x1.t()
# torch.transpose(input, dim_0, dim_1)
# 바꿀 차원을 2개 지정함
# 텐서의 내부 차원간 바꾸기
x1 = torch.FloatTensor(10, 3, 4)
# 1차원과 2차원을 서로 바꿈
x1.size(), torch.transpose(x1, 1, 2).size(), x1.transpose(1,2).size()
차원 변환 . 인덱스 지정해서 해당 인덱스끼리의 차원을 바꿈
'국비지원교육 > Python' 카테고리의 다른 글
선형회귀 with googlecolab (1) (0) | 2024.05.30 |
---|---|
pytorch - custom fuction (0) | 2024.05.29 |
pytorch 기본기 - CreateTensor with googlecolab (0) | 2024.05.28 |
pytorch 설치, numpy와 tensorflow와 비교 with google colab (0) | 2024.05.28 |
numpy 정리 (0) | 2024.05.23 |